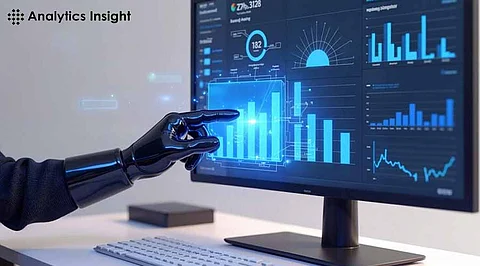
Artificial intelligence is changing the face of industries worldwide, and data analytics is no exception. AI-driven tools are increasingly being used to process, analyse, and interpret vast amounts of data in real-time, enabling businesses to gain actionable insights faster than ever. But can AI fully automate data analytics? There's only so much machines can do without people. While AI can do most of the heavy lifting, this article takes a close look at opportunities and limitations in auto-analytics and where the human factor will still always be the crucial link in the chain.
This type of AI-powered data analytics involves using artificial intelligence and its subcategories, specifically machine learning (ML) and natural language processing (NLP), to perform data analytics. AI makes it possible to automate much of the repetitive work on data cleaning, classification, and pattern recognition, and with a large dataset, this does cut down the time it takes and the effort spent in performing data analysis. It might uncover insights or points that human analysts have overlooked in the sheer volume and complexity of data.
AI analytics platforms can keep on learning from the new data. They get more efficient with use, so they are valuable assets for businesses that have extensive use of data-driven decision-making.
There are a few benefits that AI can offer to a business when it comes to streamlining processes regarding data analysis. The advantages include:
Speed and Efficiency: AI can process millions of data points in a single fraction of the time it would take for humans to analyze. It lets companies make decisions quickly with instantaneous insights.
Reduced Human Errors: Human errors are natural when handling large data processing. AI minimizes or eliminates human errors because a lot of calculations are done behind the scenes, thereby excluding bias in data interpretation.
Scalability: Human-led big data analysis does not scale well. AI-based tools scale up very easily to handle big data management without affecting performance and accuracy.
Predictive Analytics: AI is excellent in predictive modelling. Using old results and trends, AI can accurately predict the results to be experienced in the future, thereby making organizations prone to proactive decisions.
With these benefits, the question is still: Can AI take over the process of data analytics, or is there a place where human expertise is still needed?
While AI is impressive, there are quite significant challenges and limitations when it comes to fully automating data analytics.
Contextual Understanding: Though AI is very efficient in processing and analyzing data, it misses the overall context in which data are produced. Human analysts, however, take into account industry knowledge, market conditions, and external influences when interpreting data.
Data Quality Issues: AI algorithms need clean, structured data to work. If data is incomplete, biased, or poorly structured, AI models may produce results that aren't accurate. Human oversight usually ensures the data is reliable.
Ethics and Compliance Issues: AI processes data through algorithms and doesn't necessarily account for all ethics or compliance. That role falls on the judgments made by human beings ensuring analytics comply with law as well as ethics in regulated areas like healthcare and finance.
It certainly lacks creativity. Human analysts are primarily characterised by innovation and out-of-the-box thinking while explaining data, something AI can never replicate.
Human expertise is still the most important in many areas of data analytics. AI can take care of the technical aspects, but human analysts are better at interpreting data in the context of broader business strategies. Human intuition, creativity, and decision-making skills are still necessary to convert data-driven insights into actionable business strategies.
Furthermore, AI and human analysts will collaborate to generate better and more impactful insights. Here, AI serves as an auxiliary tool, supplementing human capabilities and not replacing them. Data analytics in the future will probably be hybrid: a model in which most data processing will be done by AI while humans focus on interpretation, decision-making, and ethics.
Full automation of the process is still a long way off, though AI has done much to automate various aspects of data analytics. AI systems are very efficient in handling large datasets and giving real-time insights, to improve predictive accuracy. However, these are also where the limitations lie, mainly in terms of contextual understanding and ethical judgment, and so human expertise will continue to be indispensable for a long time to come. This most likely means strategy will blend the best attributes of both worlds, automating aspects through AI systems and incorporating human-driven efforts for added depth in finding insight quality with speed.