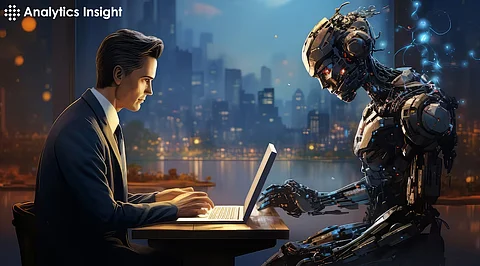
Artificial Intelligence is now all-around a transforming phenomenon in the world. Yet, commonly held is this view or notion that AI jobs are coder-focused deals. Well, in reality, there are so many AI careers available that do not require programming, making AI an accessible and available sector for all different walks of life. One can build a career in AI by focusing on specialization, complementary skills, and a deep understanding of the AI landscape without coding.
AI is a very broad field, and its branches may offer something different. While these offer great non-coding opportunities, areas such as NLP or computer vision require a great deal of technical work but also require strategic and operational support from a non-coding professional.
Roles here may involve activities such as analysis of data, project management, and production of the actual product. A specialization has to be identified quite early so that the whole skill building will be targeted towards specific demands made by a particular field. This choice of specialization also makes it easier to stay motivated and informed in real time because AI technology changes very fast.
Understanding the core principles and the tools of AI in general does not require any background in coding. It has helped professionals communicate better with technical teams on concepts around machine learning, neural networks, and natural language processing and translate business needs into AI requirements. Introductory courses, webinars, or accessible articles online are a good foundation for core AI concepts. Maintaining awareness of news and releases on AI and industry news helps build a more nuanced understanding of the issues of ethics and social impact being developed in association with AI, at least while AI continues on its trajectory towards the further de-personalization of personal life.
Not all practitioners require coding ability; they are, on the contrary, valuable in the context of data analysis, communication, project management, and business acumen. The most important analytical skills are derived based on the interpretation required by AI systems to make decisions. Tools like Tableau or Power BI also prove helpful for visualizing data and making insights that must drive AI development.
Another core competency is effective communication. Many non-coding specialists are in-house liaisons who connect the technical and non-technical teams, so they have to make complicated ideas on AI simple and understandable to stakeholders to avoid misunderstanding and bring the teams into sync with project goals. Strong skills in project management, acquired through methodologies such as Agile or software such as Asana and Jira, ensure that all the research undertaken on AI is structured well and timely.
The understanding of how AI interacts and solves business problems is the second core competency for non-coding roles. Knowledge of the applications of AI in the industry will enable one to identify areas where AI can add real value. Therefore, one can align technological possibilities with business strategies. This cuts across all roles in AI product management, consulting, and sales.
AI and data tools provide first-hand experience in performing processes of AI without coding. These user-friendly platforms include Google Cloud AI, IBM Watson, and Microsoft Azure AI. The usage of such platforms provides users with extreme powers, which can be revealed through the opportunity to test AI models and datasets. Awareness of such tools allows one to gain a superior knowledge of how AI works not through code.
Other roles are visualization tools that help in summarizing complex AI outputs for presentation of the same; possess a mastery of tools such as Tableau or Google Data Studio ability to present data-driven insights to a user. Project management software like Trello, Asana, or Jira could be used in order to keep track of AI projects, keep track and arrange tasks and to plan teamwork, thus channelling in noncoders' useful contribution toward AI projects with a well-defined workflow.
While the competition in a field is more and more coming forward, even as with the case in AI. That can be approached by interested potential candidates in product management, consulting, or policy roles, presenting case studies explaining how AI can be applied to solve some business problem, thereby demonstrating the capability to apply knowledge of AI to real-life problems.
Its credibility enhances writing articles or blog pieces on trends in AI, ethical issues about AI, or still emerging technologies. There are other channels where an opinion can be shared with respect to subjects such as AI and those include LinkedIn and Medium, in which published work will serve as a portfolio for AI thought leadership, providing places to share ideas with larger groups.
Related to AI skills, relevant certifications like data analysis or project management will be an addition to this resume. Courses that are designed for people who don't know coding, especially the ones put together by Google like "AI for Everyone" or AI courses on Coursera that discuss business aspects bring out foundational knowledge and real-world insights in AI applications. Such certification is a fantastic display of commitment to one's specialisation and expertise, so one doesn't find it very difficult to get noticed in the market.
Networking has a lot of opening doors; however, it is considerably more valuable in this fast-evolving AI field. Networking opens people's access to professionals within the field and helps them stay ahead of new trends and innovations while attending AI conferences, webinars, and meetups.
For example, AI-related groups on LinkedIn or Reddit provide a means of knowledge sharing while participating in open-source projects or online forums provides practical experience as well as strengthening connections. It may create connections to job referrals, collaborations, or mentorship for one's needs the most important factors in carving out a non-coding AI career.
This can be achieved by entry-level positions and internships, offering non-coders hands-on exposure to practice in AI through the experience acquired through data analysis, coordinating projects, and developing policies. Such steps enable professionals to gain familiarity with AI projects and workflows. Companies also offer AI-specific internships that are uncoded but focus on data interpretation, ethical analysis, or project management. Practical experience cements learning and leads towards a more profound understanding of the way AI actually functions in real life.
This is AI evolution; to stay relevant means constant learning. As AI develops, so do its tools and methodologies applied to the sector. The only way that a person would stay updated with every new trend and tool that comes forth and everything that comes with it is with the update of their skills. New courses, attendance at industry conferences, or subscription to an AI news source can be coined in words of career advancement in terms of AI.
Therefore, one can have a highly rewarding career in AI even without coding. However, that's only possible after building foundational knowledge, acquiring relevant networking skills and continuous learning; then it's a good basis for professional contributions to the field of AI. With an ever-increasing demand for noncoding roles and a growing growth phase as regards influence, it's a great time to enter and thrive in this transformative industry.