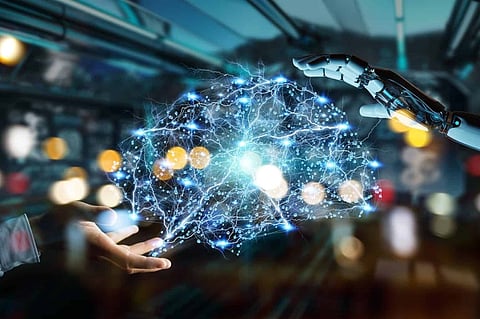
Asset-intensive organizations are pursuing digital transformation to attain operational excellence, improve KPIs, and solve concrete issues in the production and supporting process areas.
AI-based prediction models are particularly useful tools that can be deployed in complex production environments. Compared to common analytical tools, prediction models can more easily amplify correlations between different parameters in complicated production environments that generate large volumes of structured or unstructured data.
My regular talks with executives of production-intensive organizations indicate that AI use is steadily rising. This is in line with IDC's forecast that 70% of G2000 companies will use AI to develop guidance and insights for risk-based operational decision making by 2026. The figure is less than 5% today.
Please — do not be distracted by visions of a powerful "central brain" that can manage the entire organization. Typical, everyday use cases mostly leverage cognitive AI embedded in planning and scheduling tools. It is also used in quality- and maintenance-predictive models.
What delivers immediate value — and very reasonable ROI — are solutions that leverage AI-powered engines that recognize images and sound, numeric values from vibrations, temperatures, and processes. We currently see most of these use cases in pilots or isolated implementations.
From a scalability perspective, there are two main groups of digital projects that leverage AI in production areas. Each delivers value. However, they each offer different time to scale and time to accuracy.
Customized Solutions: AI-powered solutions based on complex learning processes are highly customized. They may leverage neural networks and deep learning for image recognition, or supervised learning to build predictive models.
It takes a relatively long time to fine-tune a solution to provide 90% accuracy. These are usually predictive solutions that model the behavior of material as it goes through a production process (e.g., breakage predictions for a paper belt or steel slab).
Gülsün Akhisaroglu, the global IT director for Hayat Holding, a hygiene and paper products manufacturer, told me: "It took us almost two years to achieve 90% accuracy."
This sounds like industrial scalability could be a real challenge. However, for this project, the auto-learning mode was applied — substantially accelerating progress toward 99% accuracy.
Even in highly customized models, it may be difficult to find the root causes of problems. To resolve such issues, analysts and material engineers must use intelligent solutions that show when, how, and why problems occurred.
Said CIO Akhisaroglu: "We decided to evaluate deep learning algorithms to discover any meaningful patterns. We selected eight promising algorithms out of the 92 we analyzed."
Engineers, developers, and data analysts have several digital and hardware tools and solutions at their disposal that are based on contemporary technologies. However, in many cases, these tools and solutions are inadequate. Production environments can be vastly different.
It is not a matter of simply capturing the right parameters and signals to improve the quality of outputs and the final accuracy of the model. Working conditions may vary as well. Different methods of maintaining, adjusting, and operating production assets may significantly impact the quality of model outputs. The journey toward perfection may be winding and rocky.
ROI, of course, must be extremely compelling. My experience tells me that fast solution prototyping is essential. A model's functionality should be tested quickly, in a maximum of 3–4 weeks. Lead time between the start of development and deployment of a solution (getting accurate and reliable outputs) can take months due to the learning process and model adjustment.
This is why the ideal production type for this kind of deployment is a highly asset-intensive environment — where a single stoppage can cause millions of dollars in damage.
Standardized Solutions: These are refined, highly scalable solutions based mostly on image-recognition principles. The accuracy of the final output strongly depends on the number of anomaly samples: The more samples, the more accurate the model.
For basic quality control tasks, it may take 4–6 not-OK ("NOK") samples to teach a system run via a camera positioned on the production line. This is fully sufficient in high-speed production. Theoretically, such a solution may even provide 99.99% accuracy. However, real life shows this rather theoretical value is reached only during simple quality inspection tasks.
Size and surface integrity play a big role in whether such solutions can be effectively utilized. The smaller and simpler it is, the more effective the control outputs.
Solutions that leverage AI-powered tracking and analysis of each assembly step, including cycle-time analysis, seem very promising. Such solutions can identify production anomalies and bottlenecks, improving throughput by tens of percentages.
They can also significantly speed the discovery of quality issues — in some cases reducing discovery time to minutes. Standardized solutions may easily achieve an ROI target of 1–2 years. Time to Scale and Time to Accuracy may be as little as days — or even hours.
Enterprises should have realistic expectations about leveraging AI in production, quality control, and maintenance. AI is not a miracle drug that solves every emergency. You can forget about a "supreme power" that may turn against you, like in some "war of the robots" novel.
AI can, however, provide a solid range of use cases. Your focus should be on what can be achieved by AI-powered solutions — and how much effort and money you can invest in them.
In many situations, the benefit is not only obvious KPIs (e.g., production line availability or overall equipment efficiency) — it is also secondary impacts that improve sustainability and quality, resolve problems in the production process, and boost customer satisfaction.
As always, the creation of digital silos must be avoided. To unlock the full power of data, AI-powered models must be integrated with enterprise systems like manufacturing executive systems, ERP, and advanced analytics tools. Data can be analyzed in more than one area and contextualized. Different analytics solutions can be combined to squeeze out unexpected insights.
Start now!
As you push forward, however, do not underestimate organizational, technology, and management support. CIO Akhisaroglu offers this final insight: "Looking back, we lost a lot of time in starting these pilot projects. We should have started earlier and been more proactive in collecting data from all available problem-related resources. We faced many challenges with servers, databases, and processes, but it is clear that we worked harmoniously to meet our needs quickly and effectively."
Jan Burian, Senior Director, Head of IDC Manufacturing Insights EMEA and Leader of IDC Future of Operations Europe Practice
Join our WhatsApp Channel to get the latest news, exclusives and videos on WhatsApp
_____________
Disclaimer: Analytics Insight does not provide financial advice or guidance. Also note that the cryptocurrencies mentioned/listed on the website could potentially be scams, i.e. designed to induce you to invest financial resources that may be lost forever and not be recoverable once investments are made. You are responsible for conducting your own research (DYOR) before making any investments. Read more here.