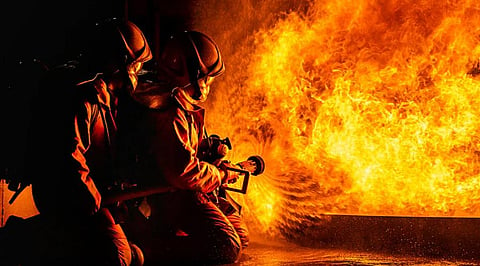
The worst flames in firefighting are the ones you don't see coming. In the midst of the chaos of a burning building, it's difficult to spot the warning signs of impending flashover — a deadly fire phenomenon in which nearly all combustible items in a room spontaneously ignite. But now AI has taken the responsibility to resolve this problem.
To date, Flashover is one of the leading causes of firefighter deaths, but new research shows that artificial intelligence could provide much-needed forewarning to first responders. Researchers at the National Institute of Standards and Technology (NIST), Hong Kong Polytechnic University, and other institutions have built a Flashover Prediction Neural Network which is also called as FlashNet to predict deadly events seconds before they occur. In a recent study that was published in Engineering Applications of Artificial Intelligence, FlashNet outperformed existing AI-based flashover forecasting tools, boasting an accuracy of up to 92.1% across more than a dozen popular residential floorplans in the US.
Flashovers tend to suddenly flare up at approximately 600 degrees Celsius (1,100 degrees Fahrenheit) and can then cause temperatures to shoot up further. To anticipate these events, existing research tools either rely on constant streams of temperature data from burning buildings or take the help of machine learning to fill in the missing data in the likely event that heat detectors succumb to high temperatures. Till now, most machine learning-based prediction tools have been designed to operate in a single, familiar environment. In reality, firefighters are not afforded such luxury. As they charge into hostile territory, they may know little to nothing about the floorplan, the location of the fire, or whether doors are open or closed.
"Our previous model only had to consider four or five rooms in one layout, but when the layout switches and you have 13 or 14 rooms, it can be a nightmare for the model," mentioned NIST mechanical engineer Wai Cheong Tam, co-first author of the new study. "For a real-world application, we believe the key is to move to a generalized model that works for many different buildings."
Join our WhatsApp Channel to get the latest news, exclusives and videos on WhatsApp
_____________
Disclaimer: Analytics Insight does not provide financial advice or guidance. Also note that the cryptocurrencies mentioned/listed on the website could potentially be scams, i.e. designed to induce you to invest financial resources that may be lost forever and not be recoverable once investments are made. You are responsible for conducting your own research (DYOR) before making any investments. Read more here.