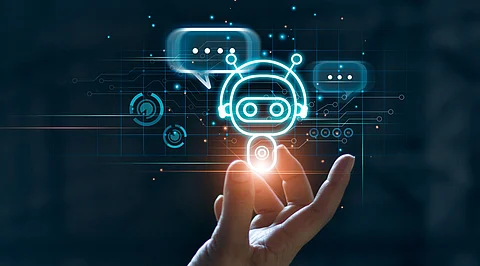
More and more companies are leveraging technological advances in machine learning, natural language processing, and other forms of artificial intelligence to provide relevant and instant recommendations to consumers. From Amazon to Netflix to REX Real Estate, firms are using AI assistant's predictions to enhance the customer experience. AI assistants are also increasingly used in the public sector to guide people to essential services. However, simply offering AI assistance won't necessarily lead to more successful transactions. There are cases when AI's suggestions and recommendations are helpful and cases when they might be detrimental. Here the question arises "when to trust an AI assistant's predictions"?
To help people better understand when to trust an AI "teammate," MIT researchers created an onboarding technique that guides humans to develop a more accurate understanding of those situations in which a machine makes correct predictions and those in which it makes incorrect AI assistant's predictions.
By showing people how the AI complements their abilities, the training technique could help humans make better decisions or come to conclusions faster when working with AI agents.
The researchers proposed a teaching phase where they gradually introduce the human to this AI model so they can, for themselves, see its weaknesses and strengths. This is done by mimicking the way the human will interact with the AI in practice, but the researchers intervene to give them feedback to help them understand each interaction they are making with the AI assistant's predictions.
This work focuses on the mental models humans build about others. If the radiologist is not sure about a case, she may ask a colleague who is an expert in a certain area. From experience and her knowledge of this colleague, she has a mental model of his strengths and weaknesses that she uses to assess his advice.
The researchers tested this teaching technique with three groups of participants. One group went through the entire onboarding technique, another group did not receive the follow-up comparison examples, and the baseline group didn't receive any teaching but could see the AI's answer in advance.
When teaching is successful, it has a significant impact. That is the takeaway here. When we can teach participants effectively, they can do better than if you gave them the answer.
But the results also show there is still a gap. Only 50 percent of those who were trained built accurate mental models of the AI assistant's predictions, and even those who did were only right 63 percent of the time. Even though they learned accurate lessons, they didn't always follow their own rules, Mozannar says.
Therefore teaching users about an AI's strengths and weaknesses is essential to producing positive human-AI joint outcomes.
Join our WhatsApp Channel to get the latest news, exclusives and videos on WhatsApp
_____________
Disclaimer: Analytics Insight does not provide financial advice or guidance. Also note that the cryptocurrencies mentioned/listed on the website could potentially be scams, i.e. designed to induce you to invest financial resources that may be lost forever and not be recoverable once investments are made. You are responsible for conducting your own research (DYOR) before making any investments. Read more here.