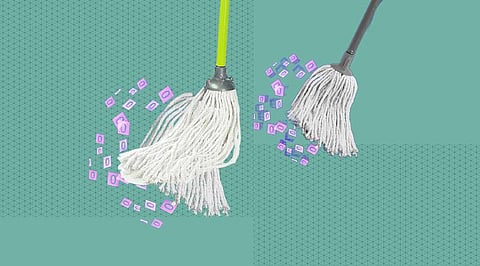
In the ever-evolving landscape of technology, Artificial Intelligence (AI) stands at the forefront of a remarkable revolution, transforming industries and reshaping our world. At the heart of this paradigm shift lies the crucial need for clean and reliable data, fueling the potential of AI to deliver unwavering results across every sector, spanning from healthcare and finance to education and beyond. In this article, we embark on a captivating journey to explore the power of harnessing pristine data in the AI era, unlocking opportunities for growth and innovation. Delving into cutting-edge tech insights and trends, we pave the way for a future where success knows no bounds.
At the core of every AI system lies data. AI algorithms learn from this data to make decisions, recognize patterns, and improve performance over time. The quality of data directly impacts the accuracy and reliability of AI models. Clean and reliable data is the foundation for cutting-edge AI technologies.
One of the primary challenges in AI is dealing with inaccurate data. Inaccuracies can stem from various sources, including human error during data entry or outdated information. AI models trained on inaccurate data can produce flawed outputs, leading to potential issues and misinformation.
Incomplete data poses another obstacle to AI systems. Gaps in the data can lead to biased models, hindering the AI's ability to make well-informed decisions. AI trained on incomplete data might only consider some relevant factors, resulting in suboptimal results.
Bias in data is a critical concern for AI applications. Biased data reflects historical inequalities and prejudices, which can perpetuate through AI systems. For instance, biased data in hiring algorithms can lead to discriminatory hiring practices.
Data cleaning, also known as data cleansing, identifies and corrects errors, inconsistencies, and inaccuracies in datasets. This step is crucial in preparing data for AI training.
Data cleaning ensures that the data used to train AI models is accurate and reliable. The AI system can learn from trustworthy information by removing errors and inconsistencies, leading to more precise outcomes.
Data cleaning helps reduce bias in AI systems by identifying and rectifying biased data points. It involves carefully examining the data for potential prejudices and making necessary adjustments to create a fairer, more inclusive AI model.
Clean data enhances the overall performance of AI algorithms. By providing high-quality data, AI models can achieve better accuracy, faster processing, and improved decision-making capabilities.
Interestingly, AI itself can play a vital role in data cleaning. AI-powered tools and algorithms can automate the data-cleaning process, making it more efficient and effective.
AI can automatically validate and cross-reference data against trusted sources, helping identify inaccuracies and inconsistencies humans might overlook.
AI algorithms can detect anomalies and outliers in datasets, indicating errors or data corruption. By flagging these anomalies, data cleaning becomes more targeted and precise.
AI can also predict missing values in datasets, replacing incomplete data with estimated values. This imputation process helps fill gaps in the data, contributing to more comprehensive AI training.
While AI brings remarkable advancements to data cleaning, human expertise remains invaluable.
Humans can provide crucial contextual understanding, especially when dealing with ambiguous data. They can discern patterns and relationships that AI might miss, ensuring a more holistic approach to data cleaning.
Data cleaning often involves ethical decisions, such as handling sensitive information or dealing with potential biases. Humans are essential in making these ethical judgments and ensuring the data remains unbiased and secure.
Human oversight helps in continuously refining AI-powered data-cleaning tools. By monitoring and validating the outputs of AI algorithms, humans can identify areas for improvement, leading to better data-cleaning processes.
Join our WhatsApp Channel to get the latest news, exclusives and videos on WhatsApp
_____________
Disclaimer: Analytics Insight does not provide financial advice or guidance. Also note that the cryptocurrencies mentioned/listed on the website could potentially be scams, i.e. designed to induce you to invest financial resources that may be lost forever and not be recoverable once investments are made. You are responsible for conducting your own research (DYOR) before making any investments. Read more here.