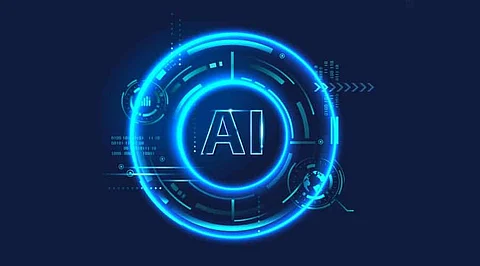
Artificial intelligence is being used by large industrial companies to analyze their long array of unstructured datasets and put them into smart use. AI is creating analytics models that are creating accurate operating strategies based on variables like pump speed or weather. To be successful in this process, the big industries must know how to create an amenable environment for AI to work properly with their big datasets.
There is a five-step approach that can be adapted to process the big datasets into smart data. First of all, the steps of the process must be outlined, along with addressing the physical and chemical changes like grinding, heating, oxidation, and polymerization. The process flow of the operation should be labeled using paint schematics or engineering drawing. In the next step, the non-standard operating regimes should be removed. A common data science approach should be used to engineer input combinations to produce new features. When combined with the sheer number of sensors available in modern plants, this demands a massive number of observations. Instead, teams should prepare the features list to include only those inputs that describe the physical process, and then they should apply deterministic equations to create features that intelligently combine sensor information.
The sensor calibrations should be addressed and a high-quality dataset should be built. The next phase of the process would be to leverage the engineering formulas to combine the sensor data in an intelligent manner.
In the next step, advanced analytic models should be overlaid on engineered data for capturing the stochastic variability. Teams should evaluate features by inspecting their importance and therefore their explanatory power. Ideally, expert-engineered features that capture, for example, the physics of the process should rank among the most important. Overall, the focus should be on creating models that drive plant improvement, as opposed to tuning a model to achieve the highest predictive accuracy. Teams should bear in mind that process data naturally exhibit high correlations. In some cases, model performance can appear excellent, but it is more important to isolate the causal components and controllable variables than to solely rely on correlations. The last step includes checking casualties and ensuring the facts that the results are physical.
The team responsible for the implementation of AI must have a variety of members from operators to data scientists, automation engineers, and process experts. Companies that are looking to implement AI generally need to rebuild their expert pipeline initially. Knowing the skills is the most important factor when it comes to choosing the perfect process expert. Planning out the model development can be a good exercise to solidify a way of working and avoid linear approaches that include exhaustively completing one stage before proceeding to the next. Later the team can decide what to invest in for the next stage.
Industrial companies are looking to AI to boost their plant operations, reduce downtime, proactively schedule maintenance, improve product quality, and so on. However, achieving operational impact from AI is not easy. To be successful, these companies will need to engineer their big data to include knowledge of the operations. The cross-functional data science teams should include employees who are capable of bridging the gap between machine learning approaches and process knowledge. Once these elements are combined with an agile way of working that advocates iterative improvement and a bias to implement findings, a true transformation can be achieved.
Join our WhatsApp Channel to get the latest news, exclusives and videos on WhatsApp
_____________
Disclaimer: Analytics Insight does not provide financial advice or guidance. Also note that the cryptocurrencies mentioned/listed on the website could potentially be scams, i.e. designed to induce you to invest financial resources that may be lost forever and not be recoverable once investments are made. You are responsible for conducting your own research (DYOR) before making any investments. Read more here.