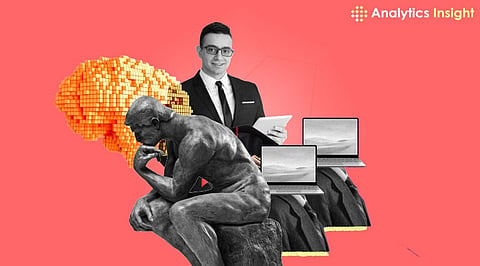
In recent years, the field of artificial intelligence (AI) has witnessed remarkable advancements, with researchers continuously pushing the boundaries of what is possible. Among the latest innovations to emerge is Double Generative AI, a groundbreaking approach that combines the power of two generative models to create highly realistic and diverse outputs.
At its core, Double Generative AI leverages the capabilities of two distinct generative models to generate synthetic data or content. The first model, known as the primary generator, is responsible for generating the initial output based on input data or random noise. This output serves as the foundation for the second model, referred to as the secondary generator, which further refines and enhances the initial output to produce a result.
The primary generator typically employs techniques such as variational autoencoders (VAEs) or generative adversarial networks (GANs) to generate realistic data samples or content. These models are trained on large datasets to learn the underlying distribution of the input data and generate outputs that closely resemble real data instances.
Once the primary generator generates an initial output, the secondary generator comes into play to perform additional processing and refinement. This secondary stage may involve techniques such as style transfer, image-to-image translation, or text-to-image synthesis to further improve the quality and diversity of the generated content.
Double Generative AI holds immense potential across various domains, ranging from computer vision and natural language processing to creative arts and entertainment. Some notable applications of Double Generative AI include:
While Double Generative AI offers unprecedented capabilities, it also poses several implications and challenges that must be addressed:
Join our WhatsApp Channel to get the latest news, exclusives and videos on WhatsApp
_____________
Disclaimer: Analytics Insight does not provide financial advice or guidance. Also note that the cryptocurrencies mentioned/listed on the website could potentially be scams, i.e. designed to induce you to invest financial resources that may be lost forever and not be recoverable once investments are made. You are responsible for conducting your own research (DYOR) before making any investments. Read more here.