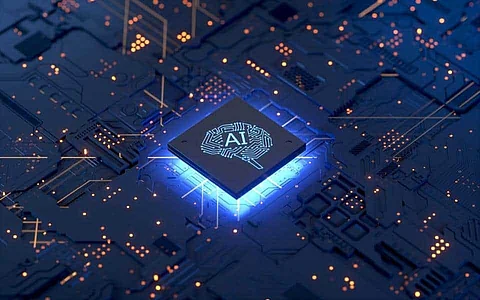
AIOps tools are picking up prominence from 2019, showing a move toward a cutting-edge IT strategy that integrates machine knowledge to better support digital transformation. Artificial intelligence for IT operations or AIOps is a class of business programming that utilizes machine learning and analytics to improve IT's ability to aggregate, analyze, and act on big data.
The purpose behind AIOps tools: find and follow up on significant insights in the environment to assist IT with running more proficient operations, settle on better choices, and support business productivity.
Despite the fact that a wide range of AI abilities, including everything from predictive analytics to natural-language processing can be applied to IT tasks, Cloud Academy mentor Guy Hummel believes they're all, by and large, utilized to help IT employees in one of two different ways. These tools are built either to automate ordinary tasks so IT administrators can concentrate more on strategic work that includes business value, or to perform tasks that are past a human's abilities.
AIOps platforms might be the most blazing ticket in big business IT at this moment, with vendors offering all types of AI-driven tools that show up with a powerful guarantee of changing IT operations. You might be enticed to adopt AIOps, yet in addition, might be overpowered by the number of the choices and by the risk of wasting time and money.
Hence, it is important to develop a strategy for optimum utilisation of AIOps tools. Let's see how we can build one.
An ultimate objective for having an AIOps tool strategy is to guarantee that the data flows freely from various IT data sources into the platforms. And afterwards, it is analysed and processed, automated workflows are set off. What's more, the whole framework works such that it adapts
and reacts to the changing data volumes. The reaction should be naturally balanced according to the data and its sensitivity and concerned chairmen should be properly informed.
Use cases must be distinguished right off the bat. The attention should be on scrutinizing the 'why' of wanted results, prioritizing use cases, and identifying the gaps between capacities, devices, skills, and processes.
With time, technologies will change, needs will move and new use cases will keep coming up, and appropriately, your ideal results will change as well. Your AIOps tools strategy, subsequently, should have the ability to address these difficulties and open up a totally different universe of conceivable outcomes.
While hardly all-in-one AIOps solutions exist today, numerous merchants offer AIOps platforms that consolidate a variety of abilities that incorporate data ingestion and management, automated pattern discovery and prediction, and root-cause failure analysis.
According to Cloud Academy's Hummel, many open-source machine learning tools are available, for example, TensorFlow and MXNet, however, most of them are broadly useful structures that require a lot of expertise to utilize. IT companies are generally happier implementing a framework that is planned explicitly for IT operations and that conceals the unpredictability of the underlying AI.
Hummel included that most of these are commercial products, albeit some of them, for example, Elastic Stack, likewise have open-source variations. However, before you begin assessing tools and vendors, you have to clearly specify key issues that your organization is hoping to solve.
You need to evaluate the ease and frequency with which data flows so you can get and send information in real-time. You need to have clear answers to questions like: How and what sort of data do I get from existing tools? How frequently would I be able to utilize it? Will I have the option to do so programmatically?
When you have relevant answers, you will be in a situation to change your data consolidation strategy and substitute your IT tools for powerful data streaming in real-time. Appraisal of data streaming abilities, in this manner, should be treated as a high-need task when you choose to build up an AIOps tools strategy.
Afterwards, utilize this insight to prepare AI and machine learning for real-time monitoring and automated response. Once AIOps frameworks are prepared with key markers of known great/known bad states, you'll have the option to monitor and alert on issues in real-time, disregarding false positives. After some time, and maybe with oversight, you'll be able to take automated actions to remediate or even forestall both known and obscure issues.
While everybody comprehends the significance of automation, we have far to go before everybody embraces it totally. In a domain where data moves and develops past the human scale, it is critical to automate all tasks and orchestrate processes.
DevOps teams are currently moving at lightning velocities to automate and arrange things and plug into the CI/CD toolchain. With the correct processes and groups, you will have the option to realize who possesses the code, what is its effect on creation, identify developer backlog and measure efficiency successfully. You should simply automate and organize the things they do across siloed tools.
Join our WhatsApp Channel to get the latest news, exclusives and videos on WhatsApp
_____________
Disclaimer: Analytics Insight does not provide financial advice or guidance. Also note that the cryptocurrencies mentioned/listed on the website could potentially be scams, i.e. designed to induce you to invest financial resources that may be lost forever and not be recoverable once investments are made. You are responsible for conducting your own research (DYOR) before making any investments. Read more here.