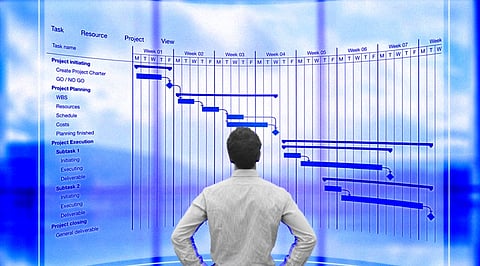
Big data initiatives are large not just in size but also in scope. Despite the fact that the majority of these projects begin with lofty goals, only a handful are successful. The vast majority of these initiatives fail. More than 85 percent of big data ventures fail. Even with the advancement of technology and advanced applications, little has changed.
According to big data specialists, adopting big data and AI efforts is difficult for enterprises. Almost every organized company is attempting to launch Machine Learning or Artificial Intelligence projects these days. They intend to get these projects into production, but it will be futile. It is still difficult for them to derive value from these ventures.
Big data projects fail due to a variety of technological issues. One of the most serious of these issues is incorrect integration. Most of the time, in order to obtain the essential insights, businesses blend contaminated data from many sources. It is difficult to connect to isolated, older systems. The cost of integration is significantly greater than the cost of the program. As a result, basic integration is one of the most difficult challenges to overcome.
If you connect every data source, nothing extraordinary will happen. The results will be nil. One of the most serious aspects of the problem is the segregated data itself. When you put data into a shared setting, it can be difficult to determine what the values mean. To enable robots to interpret the data mapped beneath, knowledge graph layers are required. Without this data, you are left with a data swamp that is useless to you. Because you would have to spend on security to prevent any future data breaches, bad integration implies big data would just be a financial burden for your firm.
Almost all of the time, technical skills fall short of business expectations. Corporations want technology to be integrated so that it can perform specific activities. The powers of AI and ML, on the other hand, are limited. Being unaware of what the project is capable of doing leads to its failure. Before you start working on a project, you should be informed of its capabilities.
Most businesses have everything they need, from resources to skills, talent to infrastructure. Nonetheless, they are unable to create an effective big data project. What causes this to happen? This occurs when the project architecture is hard and inflexible from the start. Furthermore, some businesses wait to establish a seamless architecture from the start rather than steadily developing it as the project goes.
Even if the project isn't finished and you haven't created a flawless model, you can still gain a significant amount of commercial value. Even if you just have a fraction of data to work with, you may use ML to lessen the risks.
Businesses sometimes have unrealistic expectations of the technology that is about to be implemented into their operations. Some of these assumptions are unreasonable and will be impossible to meet. Big data projects fail horribly as a result of these assumptions. While operating on big data projects, corporate leaders should set reasonable goals.
This is among the most common reasons why big data projects fail. It doesn't matter how much money you put into a project if you don't put it into production. Experts construct ML models. They are, nevertheless, left for months with nothing occurring. In the majority of cases, IT businesses lack the tools needed to construct an environment that can handle an ML model. They lack competent personnel with the knowledge to manage these models.
Wikipedia Hangs 'No Entry' Board for Bitcoin and Ethereum Donations
Top 10 Shiba Inu Price Level Predictions from Experts for 2022 and Beyond
Python Memory Error is Becoming a Concern for Developers Now
Top 10 Open-source Cybersecurity Tools for Businesses
Top 10 Harvard Courses to Learn Artificial Intelligence in 2022
Shhh! Google Might Fire You if you Question its AI Inabilities
Join our WhatsApp Channel to get the latest news, exclusives and videos on WhatsApp
_____________
Disclaimer: Analytics Insight does not provide financial advice or guidance. Also note that the cryptocurrencies mentioned/listed on the website could potentially be scams, i.e. designed to induce you to invest financial resources that may be lost forever and not be recoverable once investments are made. You are responsible for conducting your own research (DYOR) before making any investments. Read more here.