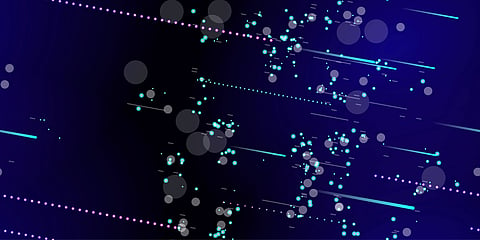
Spring is traditionally a time to commence Spring cleaning. It's also a great time for IT teams to look at how to better streamline the development and delivery of data to better support the business operations.
Here is where a DataOps approach to data integration comes into play. Similar to DevOps, DataOps is an emerging set of practices, processes and technologies for building an enhancing data and analytics pipelines to better meet the needs of the business. The promise is that this methodology will improve productivity, streamline and automate processes, increase data output and create greater collaboration across teams.
According to a survey during the recent Information Management webinar "5 Key Requirements to DataOps Success," 52 percent of respondents said they are interested in adopting a DataOps approach and an additional 41 percent are still researching the possibility. With a majority of firms interested in pursuing DataOps, it is clear that greater education is needed to assist in moving this methodology forward and break down the silos between those that own the data, the database administrators and data consumers.
Companies are operating at a fast-pace and having the right information and analysis could mean the difference between leading the market or being one of the many followers. DataOps accelerates time to insight and solves the many challenges associated with data availability.
While data managers, architects and engineers are embracing new cloud and data lake initiatives to provide a more scalable and agile infrastructure, they need to be careful to not make the same mistakes that the majority of first-generation big data projects that failed to deliver real business value and become nothing more than a large landing zone for corporate data.
For organization who operate at this speed of change, they require modern data architectures that allow for the quick use of the ever-expanding volumes of data. These infrastructures – based on hybrid and multi-cloud for greater efficiency – provide enterprises with the agility they need to compete more effectively, improve customer satisfaction and increase operational efficiencies.
When the DataOps methodology is part of these architectures, companies are empowered to support real-time data analytics and collaborative data management approaches while easing the many frustrations associated with access to analytics-ready data.
DataOps is a verb not a noun, it is something you do, not something you buy. It is a discipline that involves people, processes and enabling technology. However, as organizations shift to modern analytics and data management platforms in the cloud, you should also take a hard look at your legacy integration technology to make sure that it can support the key DataOps principles that will accelerate time to insight.
Here are five key requirements you should consider to ensure DataOps success:
1. Continuous integration– Continuous integration is foundational to these modern data platforms and to meet the needs of real-time analytics. It requires individuals to think differently about integration, it can no longer be a batch process that only happens at certain points in time and impact transactional systems. Applying technologies such as change data capture (CDC) provides a non-invasive method to catch data and metadata changes from transactional systems, relational databases and applications and stream them in real-time along to the analytic platform.
2. Universal applicability– Seeks solutions that support a broad variety of sources and targets and span multiple integration use cases. For example, you should only capture changed data once a transaction system and route that data where and when it's needed: to a data lake; or a data warehouse; or replicate to another database; etc. This provides greater efficiency and fewer moving parts.
3. Automation– It's always a challenge to find the right people and skill sets to meet the everchanging technology landscape. Automation becomes essential to the success of a DataOps initiative, from the generation of change data streams to delivery to refinement and finally the creation of analytics-ready data sets. This becomes particularly important for data lakes where heterogeneous data is coming in a variety of formats and is continuously updated with new change files.
4. Agility– Being able to embrace and adopt new technologies and implement new data pipelines is vital due to the continuous change in data formats, platforms, technology and data loads. An enterprise must be able to quickly pivot or it can be left behind the competition. Flexible integration solutions allow for IT to simply change a source or target without disrupting the entire infrastructure – providing an agile, modern infrastructure that future proofs an enterprise.
5. Trust– Trust comes from metadata and trust is absolutely essential from a business users' perspective. The last thing that you want to do is build out a Data Lake or Cloud Data Warehouse that doesn't provide data in a timely enough fashion or that provides data that can't be trusted leaving the business users blind as to where the data came from, how it was transformed and manipulated. The architecture requires a data catalog to easily find the information; data lineage to showcase where the information came from and how it has been transformed and validation to ensure that any data movement was successful.
These are the five essential items to consider when building modern data architecture and implementing a DataOps approach. Technology leaders need to be smart about future integration investments as the last generation of ETL and batch-oriented approaches are just not well suited to meet today's rapidly evolving infrastructure and business user demands.
Author: Dan Potter, VP of Product Marketing at Qlik
A 20-year marketing veteran, Dan Potter is VP of Product Marketing at Attunity – a division of Qlik. In this role, Dan is responsible for product marketing and go-to-market strategies related to modern data architectures, data integration and DataOps. Prior, he held executive positions at Datawatch, IBM, Oracle and Progress Software where he was responsible for identifying and launching solutions across a variety of emerging markets including cloud computing, real-time data streaming, federated data and e-commerce.
Join our WhatsApp Channel to get the latest news, exclusives and videos on WhatsApp
_____________
Disclaimer: Analytics Insight does not provide financial advice or guidance. Also note that the cryptocurrencies mentioned/listed on the website could potentially be scams, i.e. designed to induce you to invest financial resources that may be lost forever and not be recoverable once investments are made. You are responsible for conducting your own research (DYOR) before making any investments. Read more here.