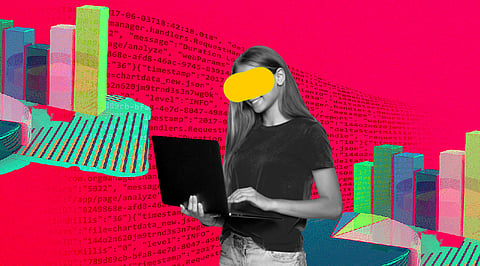
The field of data science is surging with the rising transformation in industries. For data scientists to be immaculate in their work, they have to market crucial programming languages and develop strong communication and interpersonal skills. The growing use of data has also risen the need for talented data scientists.
In this video, we present to you the top 5 most in-demand technical skills that industry hiring experts are looking for.
Data scientists must have a strong understanding of statistics, probability, linear algebra, and multivariate calculus. Key concepts like mean, median, mode, maximum likelihood indicators, standard deviation, and distributions are crucial to understanding. As a data scientist, you'll need to know about Bayes theorem, probability distribution functions, the Central Limit Theorem, expected values, standard errors, random variables, and independence.
In the field of data science, Python is the gold standard. It's a multi-purpose, object-oriented programming language that's simple to use in apps and websites and has a thriving data science community, making it a popular choice among top IT firms. The majority of data scientists use Python daily, and it has surpassed R as the most popular data science language.
SQL, Spark, Hadoop, Hive, and Pig are examples of analytical technologies that may help you extract valuable insights from data and provide effective frameworks for big data processing. In relational database management systems, SQL allows you to store, query, and change data. Spark is a processing engine that works with big, unstructured information and is straightforward to combine with Hadoop. Hadoop is an Apache Software Foundation open-source software framework for distributing massive data processing over a cluster of computer machines.
The more data a business manages, the more likely machine learning will become a part of its daily operations. Although not all data science jobs need deep learning, data engineering, or understanding of Natural Language Processing, if you want to deal with large data, you should familiarize yourself with terminologies like k-nearest neighbors, random forests, and ensemble techniques.
After gathering data from a variety of sources, you'll almost certainly come across some sloppy data that has to be cleaned up. Data wrangling is based on coding languages and aids in the correction of data flaws such as missing information, string formatting, and date formatting.
Data scientists have to create a strong foundation in these fields. With the rising demand, there is rising competition. Hence, candidates have to develop both their technical and non-technical skills.
Join our WhatsApp Channel to get the latest news, exclusives and videos on WhatsApp
_____________
Disclaimer: Analytics Insight does not provide financial advice or guidance. Also note that the cryptocurrencies mentioned/listed on the website could potentially be scams, i.e. designed to induce you to invest financial resources that may be lost forever and not be recoverable once investments are made. You are responsible for conducting your own research (DYOR) before making any investments. Read more here.