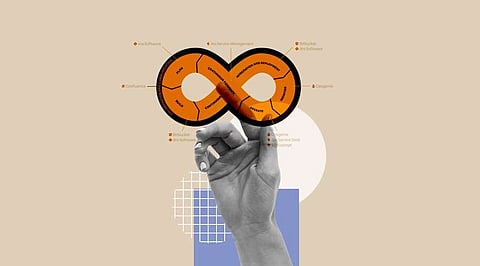
In today's data-driven world, organizations are increasingly recognizing external data value sources to bolster their decision-making processes and gain competitive advantages. However, incorporating external data can be a complex endeavour, often requiring a comprehensive approach that ensures the data's accuracy, relevance, and timeliness. This is where DataOps comes into play—a set of principles and practices that streamline the process of collecting, managing, and utilizing data. In this article, we will delve into three essential DataOps principles that can help enhance the value of external data swiftly and effectively.
A fundamental principle of DataOps is the establishment of a collaborative data ecosystem that bridges the gap between different teams and departments. In the context of external data, this means fostering collaboration not only within the organization but also with external data providers.
Start by identifying the key stakeholders involved in the external data integration process, such as data scientists, analysts, domain experts, and IT professionals. Create a cross-functional team that brings together individuals with diverse skills and perspectives. This team should work collectively to define the data requirements, quality standards, and business objectives.
Furthermore, establish strong relationships with external data providers. Engage in open communication to ensure a clear understanding of the data's structure, format, and update frequency. Regularly communicate your organization's data needs and provide feedback on the quality and relevance of the data received. By fostering collaboration both internally and externally, you can ensure that the external data is aligned with your business goals and effectively enhances decision-making processes.
The value of external data heavily depends on its quality. Poor-quality data can lead to erroneous insights and misguided decisions. Therefore, implementing robust data quality assurance practices is paramount.
Begin by conducting thorough data profiling and validation processes on the external data. Identify missing values, inconsistencies, and anomalies that might affect the accuracy of your analyses. Create data quality benchmarks and establish automated validation routines that check the data for conformity with these benchmarks. Implement data cleansing techniques to rectify issues and maintain data integrity.
Additionally, implement data lineage and auditing mechanisms to track the journey of external data from its source to its usage within your organization. This not only helps in maintaining transparency but also assists in identifying any discrepancies that might arise during the data integration process.
Traditional data integration approaches often involve long development cycles and extensive planning. DataOps encourages an agile and iterative approach to data integration that enables organizations to quickly adapt to changing data requirements and business needs.
Apply agile principles to your external data integration projects. Break down the integration process into smaller, manageable tasks or user stories. Prioritize these tasks based on their impact and feasibility, and create a continuous delivery pipeline that allows for frequent updates to the integrated data.
Automate as much of the data integration process as possible. Implement tools and technologies that facilitate the extraction, transformation, and loading (ETL) of external data into your existing systems. This reduces the manual effort required and ensures a more streamlined and efficient data integration process.
By following these DataOps principles—building a collaborative data ecosystem, ensuring data quality, and adopting an agile data integration approach—you can enhance the value of external data swiftly and effectively. Remember that data integration is an ongoing process, and continuous monitoring and improvement are essential to keep pace with the dynamic nature of external data sources.
Join our WhatsApp Channel to get the latest news, exclusives and videos on WhatsApp
_____________
Disclaimer: Analytics Insight does not provide financial advice or guidance. Also note that the cryptocurrencies mentioned/listed on the website could potentially be scams, i.e. designed to induce you to invest financial resources that may be lost forever and not be recoverable once investments are made. You are responsible for conducting your own research (DYOR) before making any investments. Read more here.