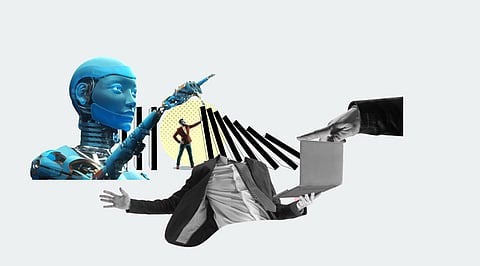
Artificial Intelligence is expanding day by day and with this expansion and adoption of AI Models it is easy for people to make blunders in AI models. The article enlists 10 major blunders to prevent when building an AI model. Some common mistakes when building an AI Model include Biased Data, and not diversifying data to name a few. The article touches upon such few AI Model Blunders.
Companies frequently encounter biased data when developing AI systems.
The AI model will promote societal biases if the training data is skewed.
Significant repercussions and unfair or discriminating outcomes may result from this.
Failure to employ a varied variety of data while training AI models is a common error made by organizations.
This can produce skewed results.
Organizations must make sure that the data used to train AI models is representative, diverse, and reflective of a range of perspectives and experiences to prevent this.
AI firms use artificial or lab-generated situations and search for "exhaustive data" for training.
The true issue is to get through it because noise and distorted data are present everywhere.
Predictions are made by machine learning algorithms, not decisions.
Because machine learning is difficult to comprehend, results can be correct but appear incorrect or incorrect but appear correct. As a result, it is impossible to determine the reasoning behind an answer.
While training AI models, organizations frequently fail to appropriately describe and validate their goals.
Without clear objectives, it might be difficult to assess an AI model's success, which can lead to subpar results or unanticipated outcomes.
The data that an organization's models are trained on starts to change as it expands into new areas, nations, and business lines based on the data that its users are presently inputting.
Iterative training of an AI model necessitates the collection of high-quality, sample data, which demands careful attention.
The correct questions are frequently not taught to organizations' AI models, or the models are not actionably integrated into processes.
Similar to how creating business analytics frequently yields a dashboard that receives little notice, trained AI models are only helpful when they are foretelling events that are significant to employees or clients.
Newcomers frequently make errors when using this fascinating technology.
One fits too tightly.
To put it simply, they overtrain the model on a specific set of inputs, and any shift makes the model rigid and narrow, not accurately reflecting the training data.
The predictions of an AI model will also include incorrect behaviors if it was taught on incorrect data.
It is crucial to make sure that the data accurately depicts both good and bad conduct when working with AI and ML applications for security and to prevent data breaches.
Most businesses struggle to create complete AI options.
They must comprehend how decision-makers work today, what information is required to make better predictions, and how model management processes input.
Join our WhatsApp Channel to get the latest news, exclusives and videos on WhatsApp
_____________
Disclaimer: Analytics Insight does not provide financial advice or guidance. Also note that the cryptocurrencies mentioned/listed on the website could potentially be scams, i.e. designed to induce you to invest financial resources that may be lost forever and not be recoverable once investments are made. You are responsible for conducting your own research (DYOR) before making any investments. Read more here.