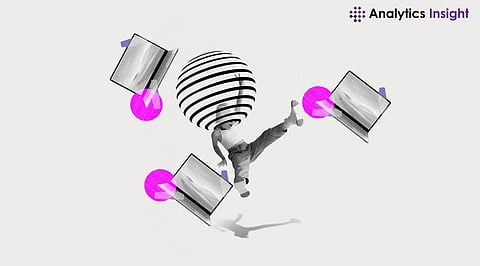
Data science is a dynamic field whose trajectory and advancement are determined by a wide range of research difficulties. These difficulties, despite their variety and complexity, highlight the essential search for understanding and creativity in the field of data science. We examine ten key research areas that span a wide range of domains and disciplines that drive the investigation and development of data science in this article.
Even though deep learning has produced incredible results, research still knows very little about its underlying mechanics. It is a challenge for researchers to understand the mathematical properties of deep learning algorithms, to make sense of the factors influencing model outcomes, and to determine the boundaries of computer capability.
The necessity for causal inference goes beyond simple correlation analysis in domains including public health, social science, and economics. Methodologies that can separate causal linkages among several variables are in high demand as data scientists work with more complex datasets. The integration of extensive data and the investigation of complex causal pathways, along with other advancements in causal inference approaches, mark the beginning of a new age in data-driven research.
Data is valuable not only in terms of quantity but also in terms of rarity, cost, and specificity. Diverse forms of priceless data present special opportunities and challenges for data science study, ranging from expensive scientific databases to artisanal knowledge stores. To meet the unique requirements of every type of data, customized approaches and algorithms are required, which consider different goals and situations.
The integration of diverse data sources holds potential to improve domain knowledge in a range of domains and strengthen predictive models. Nonetheless, there are significant technological challenges in integrating disparate data streams, such as scalability, standardization, and uncertainty management. The key to realizing the full benefits of multi-source data integration lies in the development of data fusion techniques, which will enable researchers to extract useful insights from a variety of information sources.
Data scientists face a constant challenge when navigating the intricacies of noisy and incomplete data, which is made worse by the exponential expansion in data generation and gathering. Novel techniques like differential privacy and noise-tolerant inference are becoming more and more popular as academics work to uncover meaningful patterns from poor data landscapes. Finding reliable data analysis frameworks continues to revolve around striking a balance between the trade-offs of accuracy, privacy, and scalability.
The increasing prevalence of artificial intelligence systems in crucial fields highlights the necessity of guaranteeing their credibility and dependability. AI decision-making has implications for human welfare and safety that are inextricably intertwined, ranging from autonomous automobiles to healthcare diagnostics. It will require interdisciplinary cooperation and ethical foresight to address the complex aspects of trustworthy AI, which will signal a paradigm shift in AI governance and accountability.
The architecture of computer systems must be reevaluated with an emphasis on data handling and processing efficiency due to the data-centric nature of modern applications. Domain-specific accelerators and distributed computing frameworks are examples of novel computing paradigms that have the potential to revolutionize data-intensive applications.
The predictive capabilities of machine learning are gaining prominence, but the initial phases of data processing still need a lot of work and effort. One critical requirement for optimizing data analysis workflows is the automation of data collecting, cleaning, and wrangling operations.
Data scientists have a challenging ethical and technical problem in maintaining individual confidentiality in the face of increased concerns over data privacy and security. Approaches like differential privacy and secure multiparty computation are viable solutions for balancing data exchange with privacy protection.
Ethical issues are looming huge as data science plays a bigger and bigger role in influencing society. Researchers, legislators, and industry stakeholders must work together to address ethical concerns related to data gathering, algorithmic prejudice, and responsible innovation.
Join our WhatsApp Channel to get the latest news, exclusives and videos on WhatsApp
_____________
Disclaimer: Analytics Insight does not provide financial advice or guidance. Also note that the cryptocurrencies mentioned/listed on the website could potentially be scams, i.e. designed to induce you to invest financial resources that may be lost forever and not be recoverable once investments are made. You are responsible for conducting your own research (DYOR) before making any investments. Read more here.