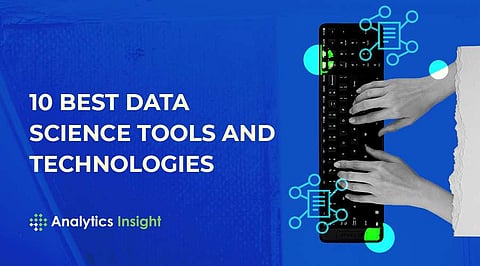
Whether you refer to it as business decision-making, planning, or forecasting for the future, data science has become increasingly important in almost every sector of the modern economy. Everything falls under the innovation and patterns that we're going ahead with. In the world of digital technology in 2022, we have a lot of data and are using a variety of tools and methods to make it useful for a variety of purposes. On the off chance that you'd discuss any famous innovation, it would be "Data Science" as it were.
To do certain things, you need to know how to use a variety of tools and any of the programming languages in data science. Even if you're willing to dig a little deeper, there are approximately 5,24,000 jobs worldwide and more than 38,000 in India right now. Based on these figures, it is necessary to stay up to date on the most data science tools and data science technologies because there is a growing demand for data scientists in almost every industry.
In recent years, Python has been by far the most widely used programming language among data scientists. In the Kaggle overview, 86.7% of information researchers said that they use Python, which was over two times the second most famous reaction. Since Python is relatively straightforward to learn, it is simple for people with no prior experience with programming to read and write Python code. A significant number of the most famous information science devices are either written in Python or exceptionally viable with Python.
TensorFlow is an open-source machine learning application development library developed by Google. Giving clients a huge range of assets and instruments, TensorFlow is notable for empowering AI designers to construct enormous and exceptionally complex brain organizations. Additionally, TensorFlow's software libraries include a large number of pre-written models to assist with specific tasks and are highly compatible with Python.
Apache Hadoop is an open-source framework for processing and storing enormous amounts of data that is extremely popular for "big data" repositories. Big data tasks are distributed across computing clusters in the way that Hadoop works. This is crucial because it makes it possible for a company's big data systems to function in a way that is both scalable and economical.
The R programming language is generally utilized for information science, all the more explicitly for measurable demonstrating and investigation. Besides Python, it's presumably the main language to be aware of for anybody working in information examination. R and Python are used by data scientists for a lot of the same things, but there are a few key differences. R places a greater emphasis on the statistical aspects of data science than Python does.
Perhaps of the most generally utilized datum perception apparatuses among information researchers, Salesforce's Scene can investigate a lot of both organized and unstructured information. It can then take the information it breaks down and convert it into various accommodating perceptions including intuitive diagrams, outlines, and guides. Tableau's ability to connect to a wide range of data sources is what makes it so useful.
SAS Viya was designed specifically for data analysis, making it one of the most complete platforms available for data management and analysis. It is one of the most well-known factual examination apparatuses among huge organizations and associations, because of its incredible dependability, security, and capacity to work with enormous informational indexes. In addition, SAS integrates with numerous well-known programming languages and tools to provide data scientists with extensive libraries and tools for data modeling.
Although the ubiquitous spreadsheet program may not be the first tool that comes to mind when you think of data science, it is one of the tools that data scientists use most frequently for data processing, data visualization, data cleaning, and calculation. Additionally, it is simple to pair with SQL for faster data analysis.
While unstructured information stores get a ton of press, information researchers accomplish a lot of work with organized information that dwells in conventional data sets. Additionally, they frequently rely on SQL (Structural Query Language) when attempting to access that data.
A large number of them are questioning information from SQL-based data sets like MySQL, PostgreSQL, SQL Server, and SQLite, yet you can likewise utilize SQL with huge information instruments like Flash and Hadoop.
DataRobot utilizes man-made brainpower and AI to help inform clients with an information display. It truly has something for everyone and aims to democratize the data modeling procedure. Business analysts with little programming experience can build sophisticated predictive models thanks to the platform's ease of use and lack of requirements for programming or machine learning.
Trifacta is a well-known information science instrument that can accelerate the course of information fighting and readiness. In a process that would otherwise take a very long time, Trifacta quickly transforms raw data into a format that data scientists can use for actual analysis. Trifacta works by automatically transforming raw data sets after combing through them to find possible changes.
Join our WhatsApp Channel to get the latest news, exclusives and videos on WhatsApp
_____________
Disclaimer: Analytics Insight does not provide financial advice or guidance. Also note that the cryptocurrencies mentioned/listed on the website could potentially be scams, i.e. designed to induce you to invest financial resources that may be lost forever and not be recoverable once investments are made. You are responsible for conducting your own research (DYOR) before making any investments. Read more here.